Determinación del número mínimo de animales al comparar las medias de tratamiento mediante análisis de potencia
Determination of minimum number of animals in comparing treatment means by power analysis
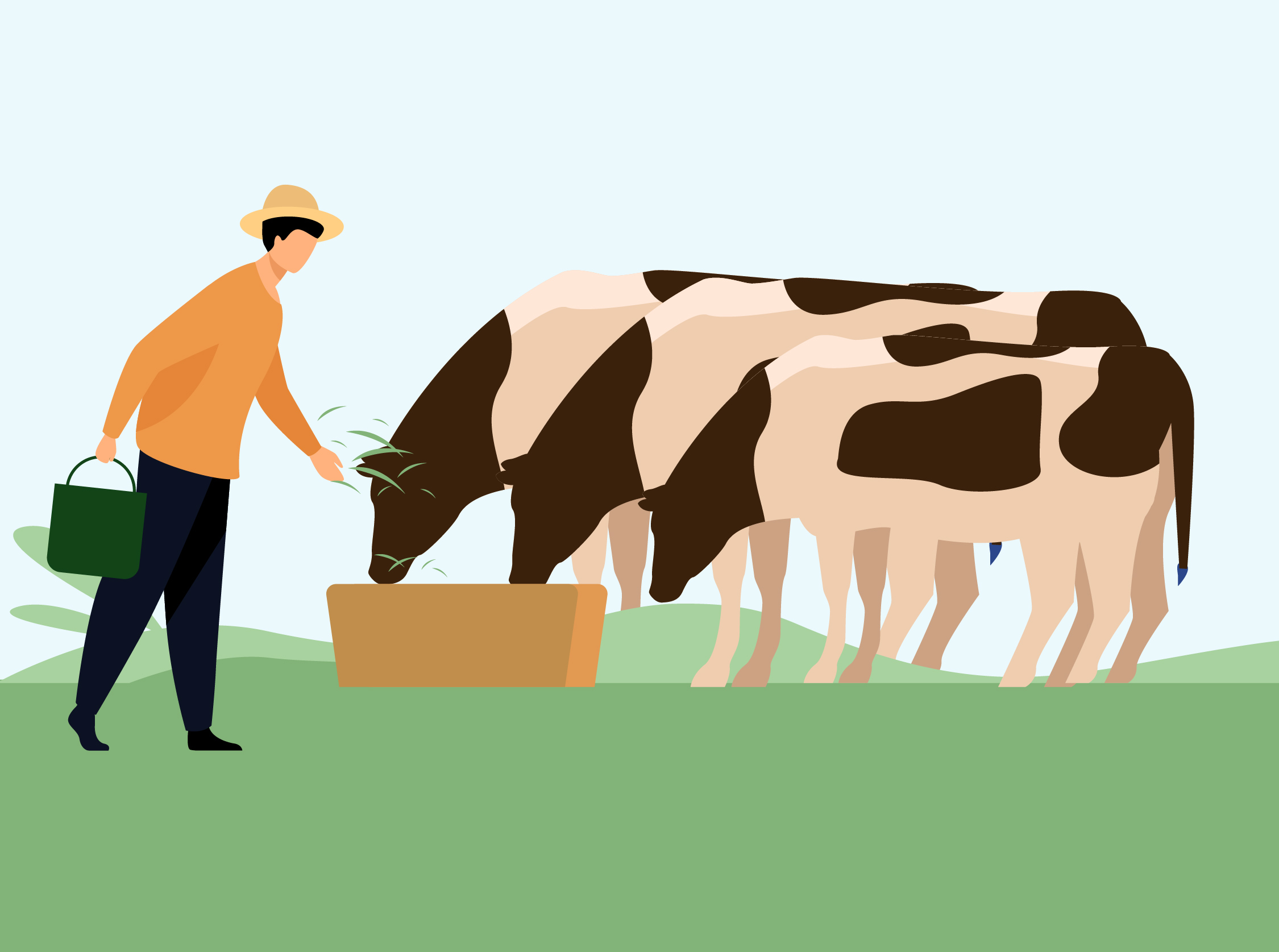

Esta obra está bajo una licencia internacional Creative Commons Atribución-NoComercial-CompartirIgual 4.0.
Mostrar biografía de los autores
Objetivo. El propósito de este estudio fue determinar el número mínimo de animales (tamaño mínimo de la muestra) en comparaciones de tratamientos con diferentes tamaños de efecto (0.25-2.0), el número de tratamientos (2-7) y la potencia de la prueba (80- 95%). Además, se desarrollaron ecuaciones de regresión lineal, cuadrática y cúbica que estiman el tamaño mínimo de muestra que debe usarse en las comparaciones de tratamientos. Materiales y métodos. Dentro del alcance de esta investigación, se utilizó la ganancia media diaria (GMD) de los experimentos con ganado de engorde a corral realizados en la Universidad Estatal de Iowa con un total de 1283 novillos. La potencia de la prueba se calculó después de que se tomaron muestras aleatorias de los datos de GMD y se establecieron las diferencias entre los tratamientos en términos de desviación estándar. Este proceso se repitió 1000 veces mediante una macro escrita en el programa del paquete de Minitab en la cantidad de tratamientos y niveles de potencia a comparar. Resultados. Se encontró que las ecuaciones de regresión cúbica dieron resultados más fiables que las demás. Como resultado, después de determinar el número de tratamientos, la potencia de la prueba y el tamaño del efecto, se puede determinar fácilmente un número suficiente de unidades experimentales utilizando las ecuaciones de estimación creadas sin análisis de potencia. Conclusiones. De esta manera, se pueden prevenir los gastos excesivos de dinero y las pérdidas financieras en estudios científicos y se puede brindar la oportunidad de encontrar financiamiento más fácilmente.
Visitas del artículo 348 | Visitas PDF
Descargas
- Akobeng AK. Understanding type I and type II errors, statistical power and sample size. Acta Paediatrica. 2016; 105(6):605-609. https://doi.org/10.1111/apa.13384
- Greenland S, Senn SJ, Rothman KJ, Carlin JB, Poole, C, Goodman SN, Altman DG. Statistical tests, P values, confidence intervals, and power: a guide to misinterpretations. Eur J Epidemiol. 2016; 31(4):337-350. https://doi.org/10.1007/s10654-016-0149-3
- Cohen J. Statistical power analysis: Current Directions in Psychological Science 1992; 1(3):98-101. https://doi.org/10.1111/1467-8721.ep10768783
- Wilcox RR. ANOVA: A paradigm for low power and misleading measures of effect size?. Rev Educ Res. 1995; 65(1):51-77. https://doi.org/10.3102/00346543065001051
- Kelley K, Rausch, JR. Sample size planning for the standardized mean difference: Accuracy in parameter estimation via narrow confidence intervals. Psychol Methods. 2006; 11(4):363–385. https://doi.org/10.1037/1082-989X.11.4.363
- Houle TT, Penzien DB, Houle CK. Statistical power and sample size estimation for headache research: An overview and power calculation tools. Headache. 2005; 45(5):414-418. https://doi.org/10.1111/j.1526-4610.2005.05092.x
- Mascha EJ, Vetter TR. Significance, errors, power, and sample size: the blocking and tackling of statistics. Anesth Analg. 2018; 126(2):691-698. https://doi.org/10.1213/ANE.0000000000002741
- Aslan E, Koşkan Ö, Altay Y. Determination of the Sample Size on Different Independent K Group Comparisons by Power Analysis. Turk Tarim Arast Derg. 2021; 8(1):34-41. https://doi.org/10.19159/tutad.792694
- Szucs D, Ioannidis JP. Sample size evolution in neuroimaging research: An evaluation of highly-cited studies (1990–2012) and of latest practices (2017–2018) in high-impact journals. NeuroImage. 2020; 221:117-164. https://doi.org/10.1016/j.neuroimage.2020.117164
- Murphy KR. Myors B. Statistical power analysis: A simple and general model for traditional and modern hypothesis tests. Second Edition (2rd ed.). Mahwah, New Jersey, USA: Lawrence Erlbaum Associates Publishers; 2004.
- Lane SP, Hennes EP. Power struggles: Estimating sample size for multilevel relationships research. J Soc Pers Relat. 2018; 35(1):7-31. https://doi.org/10.1177/0265407517710342
- Peterman RM. Statistical power analysis can improve fisheries research and management. Can J Fish Aquat Sci. 1990; 47(1):2–15. https://doi.org/10.1139/f90-001
- Fairweather PG. Statistical power and design requirements for environmental monitoring. Mar Freshw Res. 1991; 42(5):555–567. https://doi.org/10.1071/MF9910555
- Muller KE, Benignus VA. Increasing scientific power with statistical power. Neurotoxicol Teratol. 1992; 14(3):211–219. https://doi.org/10.1016/0892-0362(92)90019-7
- Taylor BL, Gerrodette T. The uses of statistical power in conservation biology: the vaquita and northern spotted owl. Conserv Biol Ser. 1993; 7(3):489–500. https://doi.org/10.1046/j.1523-1739.1993.07030489.x
- Searcy-Bernal R. Statistical power and aquacultural research. Aquaculture. 1994; 127(4):371–388. https://doi.org/10.1016/0044-8486(94)90239-9
- Thomas L, Juanes F. The importance of statistical power analysis: an example from animal behaviour. Anim. Behav. 1996; 52(4):856–859. https://doi.org/10.1006/anbe.1996.0232
- Thomas L, Retrospective power analysis. Conservation Biology. Conservation Biology. 1997; 11(1):276-280. https://www.jstor.org/stable/2387304
- Pinu FR, Beale DJ, Paten AM, Kouremenos K, Swarup S, Schirra HJ, Wishart D. Systems biology and multi-omics integration: viewpoints from the metabolomics research community. Metabolites. 2019; 9(4):76. https://doi.org/10.3390/metabo9040076
- Hoenig JM, Heisey DM. The abuse of power: the pervasive fallacy of power calculations for data analysis. Am Stat. 2001; 55(1):19-24. https://doi.org/10.1198/000313001300339897
- Faul F, Erdfelder E, Lang AG, Buchner AG. Power 3: A flexible statistical power analysis program for the social, behavioral, and biomedical sciences. Behav Res Methods. 2007; 39(2):175-191. https://doi.org/10.3758/BF03193146
- La Rosa PS, Brooks JP, Deych E, Boone EL, Edwards DJ, Wang Q, Sodergren E, Weinstock G, Shannon WD. Hypothesis testing and power calculations for taxonomic-based human microbiome data. PloS One. 2012; 7(12):e52078. https://doi.org/10.1371/journal.pone.0052078
- Fanelli D. Do pressures to publish increase scientists’ bias? An empirical support from US States Data. PloS One. 2010; 5(4):e10271. https://doi.org/10.1371/journal.pone.0010271
- Abt G, Boreham C, Davison G, Jackson R, Nevill A, Wallacea E, Williamsa M. Power, precision, and sample size estimation in sport and exercise science research. J Sports Sci. 2020; 38(17):1993-1935. https://10.1080/02640414.2020.1776002
- Lewis KP. Statistical power, sample sizes, and the software to calculate them easily. BioScience. 2006; 56(7):607-612. https://doi.org/10.1641/0006-3568(2006)56[607:SPSSAT]2.0.CO;2
- Zar JH. Biostatistical Analysis: Pearson New International Edition. New Jersey, NJ, USA: Pearson Higher Edition; 2013.
- Hartley HO. The maximum F-ratio as a short-cut test for heterogeneity of variance. Biometrika. 1950; 37(3/4):308-312. https://doi.org/10.2307/2332383
- Pearson ES, Hartley HO. Charts of the power function for analysis of variance tests, derived from the non-central F-distribution. Biometrika. 1951; 38(1/2):112-130. https://doi.org/10.2307/2332321
- Başpınar E. Gürbüz F, The power of the test in comparing samples of different sample sizes taken from binary combinations of Normal, Beta, Gamma (Chi-square) and Weibull distributions. J Agric Sci. 2000; 6(1):116-127. https://doi.org/10.1501/Tarimbil_0000000940
- Lenth RV. Statistical power calculations. J Anim Sci. 2007; 85(13):24-29. https://doi.org/10.2527/jas.2006-449
- Başpınar E, Type I error and power of the test obtained by applying Student’s t, Welch and sorted t-tests on two samples of different sample sizes taken from normal populations with different variance ratios. J Anim Sci. 2001; 7(1):151-157. https://doi.org/10.1501/Tarimbil_0000000271
- Koşkan Ö, Gürbüz F. Resampling approach and power of t-test and comparison of type I error. J Anim Prod. 2008; 49(1):29-37. https://dergipark.org.tr/en/pub/hayuretim/issue/7617/99817
- Kalaycioğlu O, Akhanli SE. The importance and main principles of power analysis in health research: Application examples on medical case studies. Turk J Public Health. 2020;. 18(1):103-112. https://doi.org/10.20518/tjph.602400
- Mewhort, DJ, A comparison of the randomization test with the F test when error is skewed. Behav Res Methods. 2005; 37(3):426-435. https://doi.org/10.3758/BF03192711