Determination of minimum number of animals in comparing treatment means by power analysis
Determinación del número mínimo de animales al comparar las medias de tratamiento mediante análisis de potencia
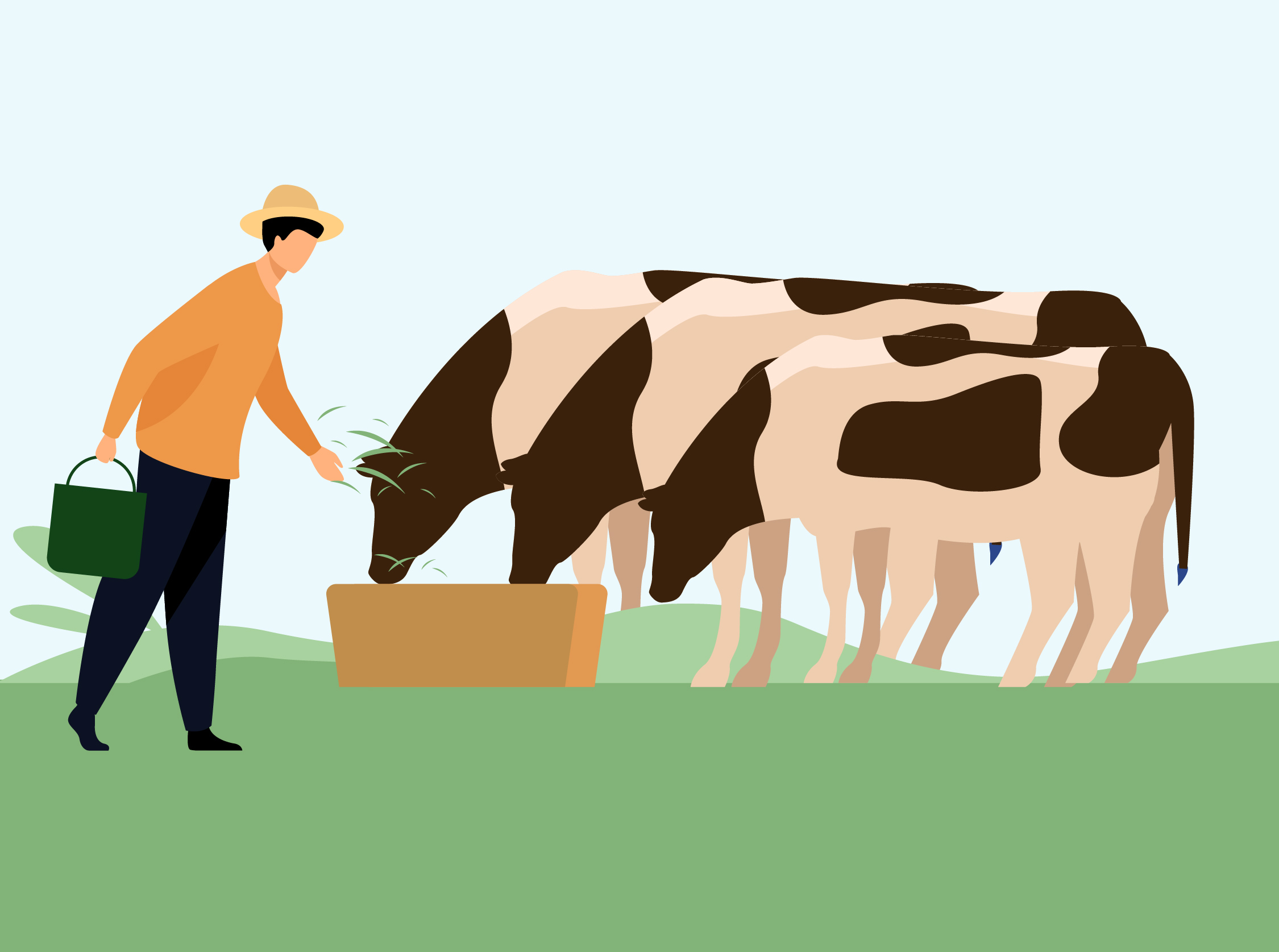

This work is licensed under a Creative Commons Attribution-NonCommercial-ShareAlike 4.0 International License.
Show authors biography
Objective. The purpose of this study was to determine the minimum number of animals (minimum sample size) in treatment comparisons with different effect sizes (0.25-2.0), the number of treatments (2-7), and the power of the test (80-95%). In addition, linear, quadratic, and cubic regressions equations that estimate the minimum sample size that should be used in treatment comparisons were developed. Materials and methods. Within the scope of this research, average daily gain (GDP) of feedlot cattle experiments conducted at Iowa State University totaling 1283 steers were used. The power of the test was calculated after random samples were taken from the GDP data and the differences between the treatments in terms of standard deviation were established. This process was iterated 1000 times via a macro written in the Minitab package program in the number of treatments and power levels to be compared. Results. It was found that the cubic regression equations gave more reliable results than others. As a result, after determining the number of treatments, the power of the test, and the effect size, a sufficient number of experimental units can be easily determined by using the estimation equations created without power analysis. Conclusions. In this way, excess money expenditure and financial loss in scientific studies can be prevented and the opportunity to find financing more easily can be provided.
Article visits 348 | PDF visits
Downloads
- Akobeng AK. Understanding type I and type II errors, statistical power and sample size. Acta Paediatrica. 2016; 105(6):605-609. https://doi.org/10.1111/apa.13384
- Greenland S, Senn SJ, Rothman KJ, Carlin JB, Poole, C, Goodman SN, Altman DG. Statistical tests, P values, confidence intervals, and power: a guide to misinterpretations. Eur J Epidemiol. 2016; 31(4):337-350. https://doi.org/10.1007/s10654-016-0149-3
- Cohen J. Statistical power analysis: Current Directions in Psychological Science 1992; 1(3):98-101. https://doi.org/10.1111/1467-8721.ep10768783
- Wilcox RR. ANOVA: A paradigm for low power and misleading measures of effect size?. Rev Educ Res. 1995; 65(1):51-77. https://doi.org/10.3102/00346543065001051
- Kelley K, Rausch, JR. Sample size planning for the standardized mean difference: Accuracy in parameter estimation via narrow confidence intervals. Psychol Methods. 2006; 11(4):363–385. https://doi.org/10.1037/1082-989X.11.4.363
- Houle TT, Penzien DB, Houle CK. Statistical power and sample size estimation for headache research: An overview and power calculation tools. Headache. 2005; 45(5):414-418. https://doi.org/10.1111/j.1526-4610.2005.05092.x
- Mascha EJ, Vetter TR. Significance, errors, power, and sample size: the blocking and tackling of statistics. Anesth Analg. 2018; 126(2):691-698. https://doi.org/10.1213/ANE.0000000000002741
- Aslan E, Koşkan Ö, Altay Y. Determination of the Sample Size on Different Independent K Group Comparisons by Power Analysis. Turk Tarim Arast Derg. 2021; 8(1):34-41. https://doi.org/10.19159/tutad.792694
- Szucs D, Ioannidis JP. Sample size evolution in neuroimaging research: An evaluation of highly-cited studies (1990–2012) and of latest practices (2017–2018) in high-impact journals. NeuroImage. 2020; 221:117-164. https://doi.org/10.1016/j.neuroimage.2020.117164
- Murphy KR. Myors B. Statistical power analysis: A simple and general model for traditional and modern hypothesis tests. Second Edition (2rd ed.). Mahwah, New Jersey, USA: Lawrence Erlbaum Associates Publishers; 2004.
- Lane SP, Hennes EP. Power struggles: Estimating sample size for multilevel relationships research. J Soc Pers Relat. 2018; 35(1):7-31. https://doi.org/10.1177/0265407517710342
- Peterman RM. Statistical power analysis can improve fisheries research and management. Can J Fish Aquat Sci. 1990; 47(1):2–15. https://doi.org/10.1139/f90-001
- Fairweather PG. Statistical power and design requirements for environmental monitoring. Mar Freshw Res. 1991; 42(5):555–567. https://doi.org/10.1071/MF9910555
- Muller KE, Benignus VA. Increasing scientific power with statistical power. Neurotoxicol Teratol. 1992; 14(3):211–219. https://doi.org/10.1016/0892-0362(92)90019-7
- Taylor BL, Gerrodette T. The uses of statistical power in conservation biology: the vaquita and northern spotted owl. Conserv Biol Ser. 1993; 7(3):489–500. https://doi.org/10.1046/j.1523-1739.1993.07030489.x
- Searcy-Bernal R. Statistical power and aquacultural research. Aquaculture. 1994; 127(4):371–388. https://doi.org/10.1016/0044-8486(94)90239-9
- Thomas L, Juanes F. The importance of statistical power analysis: an example from animal behaviour. Anim. Behav. 1996; 52(4):856–859. https://doi.org/10.1006/anbe.1996.0232
- Thomas L, Retrospective power analysis. Conservation Biology. Conservation Biology. 1997; 11(1):276-280. https://www.jstor.org/stable/2387304
- Pinu FR, Beale DJ, Paten AM, Kouremenos K, Swarup S, Schirra HJ, Wishart D. Systems biology and multi-omics integration: viewpoints from the metabolomics research community. Metabolites. 2019; 9(4):76. https://doi.org/10.3390/metabo9040076
- Hoenig JM, Heisey DM. The abuse of power: the pervasive fallacy of power calculations for data analysis. Am Stat. 2001; 55(1):19-24. https://doi.org/10.1198/000313001300339897
- Faul F, Erdfelder E, Lang AG, Buchner AG. Power 3: A flexible statistical power analysis program for the social, behavioral, and biomedical sciences. Behav Res Methods. 2007; 39(2):175-191. https://doi.org/10.3758/BF03193146
- La Rosa PS, Brooks JP, Deych E, Boone EL, Edwards DJ, Wang Q, Sodergren E, Weinstock G, Shannon WD. Hypothesis testing and power calculations for taxonomic-based human microbiome data. PloS One. 2012; 7(12):e52078. https://doi.org/10.1371/journal.pone.0052078
- Fanelli D. Do pressures to publish increase scientists’ bias? An empirical support from US States Data. PloS One. 2010; 5(4):e10271. https://doi.org/10.1371/journal.pone.0010271
- Abt G, Boreham C, Davison G, Jackson R, Nevill A, Wallacea E, Williamsa M. Power, precision, and sample size estimation in sport and exercise science research. J Sports Sci. 2020; 38(17):1993-1935. https://10.1080/02640414.2020.1776002
- Lewis KP. Statistical power, sample sizes, and the software to calculate them easily. BioScience. 2006; 56(7):607-612. https://doi.org/10.1641/0006-3568(2006)56[607:SPSSAT]2.0.CO;2
- Zar JH. Biostatistical Analysis: Pearson New International Edition. New Jersey, NJ, USA: Pearson Higher Edition; 2013.
- Hartley HO. The maximum F-ratio as a short-cut test for heterogeneity of variance. Biometrika. 1950; 37(3/4):308-312. https://doi.org/10.2307/2332383
- Pearson ES, Hartley HO. Charts of the power function for analysis of variance tests, derived from the non-central F-distribution. Biometrika. 1951; 38(1/2):112-130. https://doi.org/10.2307/2332321
- Başpınar E. Gürbüz F, The power of the test in comparing samples of different sample sizes taken from binary combinations of Normal, Beta, Gamma (Chi-square) and Weibull distributions. J Agric Sci. 2000; 6(1):116-127. https://doi.org/10.1501/Tarimbil_0000000940
- Lenth RV. Statistical power calculations. J Anim Sci. 2007; 85(13):24-29. https://doi.org/10.2527/jas.2006-449
- Başpınar E, Type I error and power of the test obtained by applying Student’s t, Welch and sorted t-tests on two samples of different sample sizes taken from normal populations with different variance ratios. J Anim Sci. 2001; 7(1):151-157. https://doi.org/10.1501/Tarimbil_0000000271
- Koşkan Ö, Gürbüz F. Resampling approach and power of t-test and comparison of type I error. J Anim Prod. 2008; 49(1):29-37. https://dergipark.org.tr/en/pub/hayuretim/issue/7617/99817
- Kalaycioğlu O, Akhanli SE. The importance and main principles of power analysis in health research: Application examples on medical case studies. Turk J Public Health. 2020;. 18(1):103-112. https://doi.org/10.20518/tjph.602400
- Mewhort, DJ, A comparison of the randomization test with the F test when error is skewed. Behav Res Methods. 2005; 37(3):426-435. https://doi.org/10.3758/BF03192711